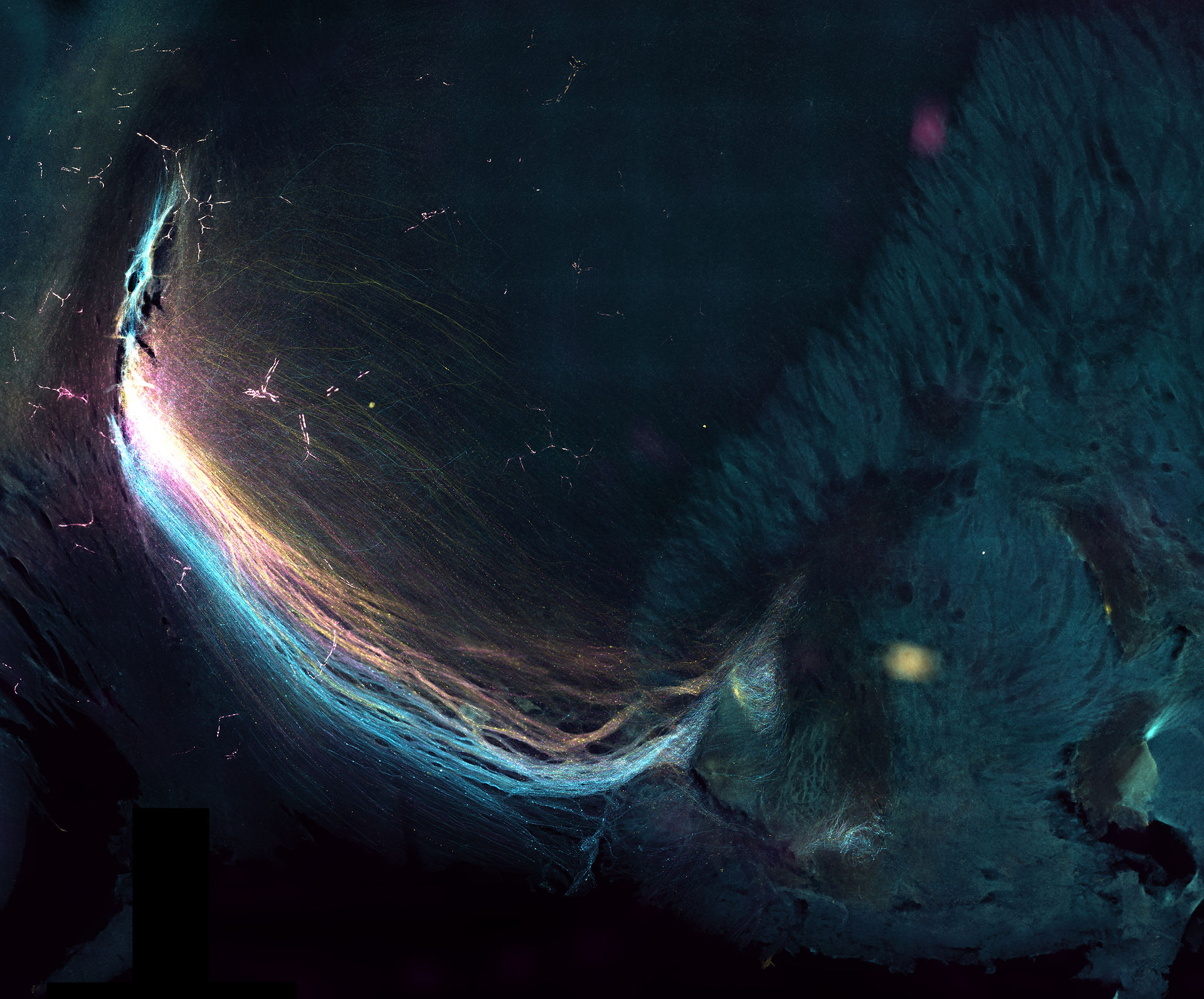
Striking a Pose in the Cortex, by Bartul Mimica
Have you ever watched a top tennis player serve? Serving a tennis ball is an acquired skill, which, to be executed properly, requires a sequence of specific poses to be strung together precisely over a brief period of time. Positioning of the arms, eyes, head, back, hips, knees and feet changes quickly, but their respective postural relationships are important at any given moment (Fig. 1). One might wonder whether, and if so how, these states of the body are represented in the brain.
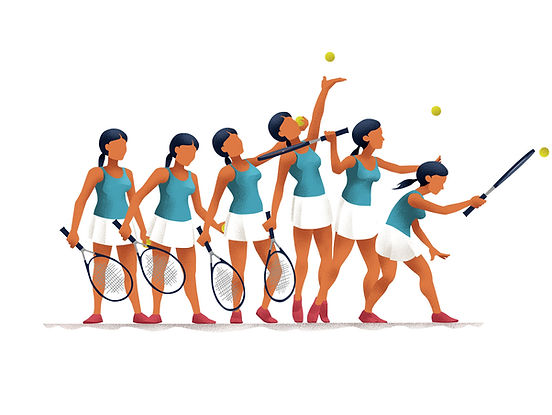
illustration by Goran Radosevic
Figure 1. A schematic of a sequence of poses required to execute the serve of a tennis ball
This line of enquiry is complementary to the general quest of understanding how behaviour is encoded in neuronal activity. But why is behaviour important? To put it simply, without behaviour - we wouldn’t know other minds existed. Even with all sensory systems intact, if behaviour is removed from the equation, we become oblivious to all psychological reality associated with others. Since bodily action is essential in projecting our inner life onto our surroundings, one of the main missions of the field of neuroscience is to elucidate this important feature of our nervous systems. The last couple of years have seen repeated calls to modernize ethologically underdeveloped paradigms in mammals and, of late, fruitful progress has been made to quantify behaviour more precisely.
Here at the Kavli Institute for Systems Neuroscience in Norway, we are curious to learn the specifics of rodent behaviour. So far, rats have been used successfully to uncover robust inter-regional networks underlying functions such as memory and spatial navigation. But rats are creatures that have a rich behavioural repertoire as well. Much like tennis players going through specific postural sequences when they serve, rats also tend to employ a recognizable succession of postures as they, for example, explore the edges of an environment (Fig. 2).

illustration by Goran Radosevic
Figure 2. A schematic of a rat rearing, scanning and coming down from a rear
However, since researchers were previously primarily interested in how neurons respond to 2-D spatial locations or head direction, it was sufficient to track only the heads of the animals with one overhead camera, effectively reducing the animals to direction-informative dots in 2-D space. In our latest study, we have attempted to correct for this under-representation of behaviour by tracking the rats’ heads and backs with multiple cameras in 3-D. You can check how these approaches measure up against each other in Fig. 3 - on the left is an overhead view of a rat head in 2-D during a recording session, and on the right the same data but with a finer 3-D representation of the head and back.
Figure 3. Comparison of 2-D and 3-D tracking on the same dataset (click to play video)
Having enhanced the level of acquired detail by upgrading our tracking method, we paired the behavioural output with single-cell recordings in order to resolve which specific features drove the neurons to respond most strongly. In this study, we were limiting our interest to two brain regions, namely the posterior parietal cortex (PPC) and the premotor cortex (M2 or AGm), as both had been implicated in a host of functions, like decision making, multi-sensory integration and movement planning, to name a few (Fig. 4). Neurons in higher-order cortices rarely lend themselves to an easy read-out, so the challenge at hand was to see whether we could use our data to extract a simple account of their tuning properties, from an endless reservoir of behavioural complexity.
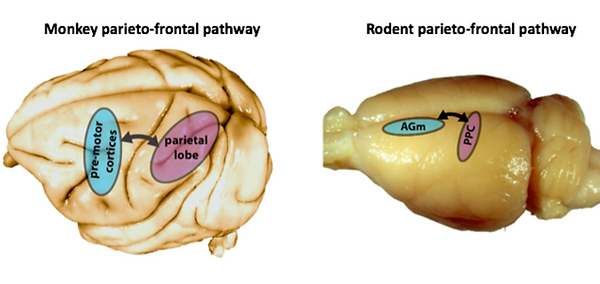
Figure 4. Anatomical locations of the posterior parietal (PPC) and premotor (AGm) cortices on the surface of primate and rodent brains, respectively
We repeatedly recorded single neurons from rats freely moving in an open arena, foraging for cookie crumbs. Our main findings were rather surprising. Most of the recorded cells’ activity properties were best accounted for by various postural features of the head, back and neck, sometimes even jointly, instead of features like spatial location, body speed or movement. This was true for both brain regions. To get a flavour for the clarity of our findings, take a look at the Fig. 5. It shows the tuning curves (i.e. activity response profiles) of three different neurons for three different postural features of the head, back and neck (as depicted by the cartoon rat). Not only are they quite striking and regular, but they are also stable, as they look quite similar from one recording session to another. This is true even when rats had to traverse the same environment in darkness, diminishing the likelihood that our findings were an artefact of visual phenomena.
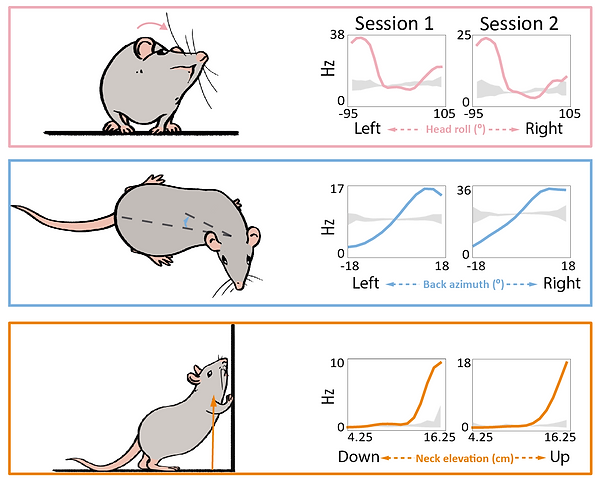
Figure 5. Tuning curves of three different neurons, each for a given feature of the head, back or neck
Moreover, the cortical representation of posture seems to be organized in an energetically efficient manner. To convey this simply, neurons tend to fire more during postures which are less visited. Inversely, cellular energy is generally conserved when rats are doing what they do most of the time – forage for food crumbs on all fours, with their head pitched down to the floor. There is another related finding, which makes this idea more appealing and elegant. When we used the combined activity of all the neurons in one recording session to reconstruct or decode the postures the animal was in, we were remarkably successful. In Fig. 6, you can see a variety of executed postures in a short sequence from the session (left). The performance of our decoder (right) in a posture-map (i.e. a low-dimensional embedding of the raw postural features) is colour-coded such that hotter colours represent higher probabilities the animal is in a given posture and this is matched to the real location of the rat in a posture map (green cross). Importantly, the decoding worked better in the portion of the posture map which was occupied the most, but was least represented in terms of informative neural activity. This is perhaps relevant, because it shows that other brain regions receiving this input would be able to reconstruct what the animal was doing, even though the network feeding them the information is relatively idle.
Figure 6. Reconstruction of ongoing posture with neural activity
(click to play video)
To sum up, higher-order cortices seem to care about postural signals. The code is orderly and pervasive across animals and recording conditions. This study has unleashed a lot of questions in our minds, questions we would be curious to pursue further. Why posture? What other brain regions are loaded with this signal? Where is the signal coming from? Do other species exhibit the same phenomenology? If you would like to learn more about the subject or other interesting findings, please read the paper, and feel free to contact me (bartul.mimica@ntnu.no; Twitter: @BartulEm) for additional details or discussion.
_______________________________